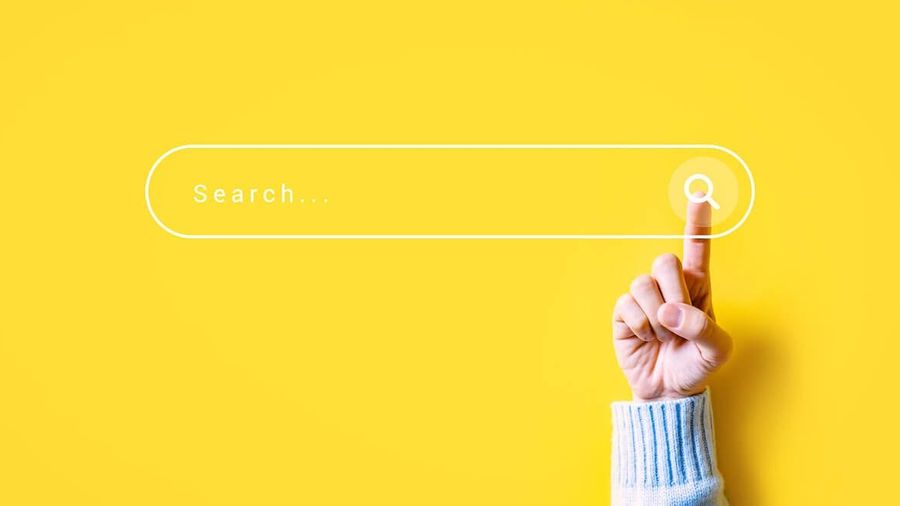
· 8 min read
AI Search engine
Search functionality is fundamental to virtually every website, application, social media platform, and e-commerce site, serving as the primary means by which we navigate and access information online. How advanced AI search can be leveraged in organizations?
Search functionality is fundamental to virtually every website, application, social media platform, and e-commerce site, serving as the primary means by which we navigate and access information online.
When we think about search, Google often comes to mind first. It's nearly synonymous with internet search, and for most users, Google's capabilities meet their needs effectively.
However, the landscape changes when we consider specialized fields of knowledge, proprietary documents, and datasets that are inaccessible to Google's indexing bots. The same relates to searching through specified knowledge bases - internal company information, websites, user applications etc. In these realms, the typical search experience fall short, highlighting the need for more tailored and sophisticated search solutions.
The Limitations of Traditional Search Engines
General vs. Specialized Search Needs
Traditional search engines like Google search have been engineered to cater to the broadest possible audience. They excel at scanning the vast expanse of the public internet to return general information that is likely to satisfy common queries. This broad optimization is achieved through complex algorithms that prioritize popular, authoritative content, making them incredibly effective for everyday searches ranging from news articles and commercial websites to general knowledge and public data.
General Search Characteristics
- Wide Indexing: Traditional search engines index billions of pages to ensure a comprehensive search experience for general topics. This extensive indexing helps users find relevant information across a broad range of subjects.
- Keyword-Based and NLP Searching: While traditional search engines initially relied heavily on keyword matching, modern engines like Google utilize Natural Language Processing (NLP) methods. NLP allows for a more sophisticated understanding of user queries, capturing nuances and intent that keyword-based searching might miss. This results in more accurate and contextually relevant search results.
- SEO and Popularity Metrics: Search results are often ranked based on Search Engine Optimization (SEO) strategies and the popularity of content. This ranking system is effective for general interests but can be less suitable for academic or niche inquiries where the most popular content may not be the most relevant or authoritative.
- Paid Ads: Paid advertisements are often displayed alongside search results. While they can provide useful information and options, they can also clutter the search results and potentially lead to a less straightforward search experience. These ads are typically marked as sponsored content but can still affect the overall quality and neutrality of search results.
However, when it comes to specialized needs — such as academic research, proprietary corporate data, or specific professional knowledge bases — these search engines often fall short. Specialized search demands go beyond what general search engines can provide in terms of depth, specificity, and privacy.
Specialized Search Requirements
- Contextual Understanding: Professionals in fields like law, medicine or finance need search tools that understand the context and jargon specific to their industry. These users benefit from AI-enhanced search engines that can interpret complex queries and deliver precise results.
- Data Sensitivity: In corporate environments, search tools must navigate private databases securely, respecting access permissions and data protection laws, which is beyond the capabilities of general search engines.
- Custom Indexing: Unlike the public internet, specialized databases are not always structured in a way that traditional search engines can easily index. Custom solutions are required to traverse these unique data structures.
AI search engines address these specialized needs by incorporating advanced technologies such as natural language processing (NLP), which allows the search engine natural language understanding in a way that mimics human comprehension. This capability enables AI search tools to discern intent and context, providing more accurate and relevant results for specialized queries. Additionally, machine learning models can be trained on specific datasets to refine their accuracy and effectiveness continually, tailoring their responses to the specialized needs of their users.
Furthermore, AI search engines can be programmed to adhere to strict privacy and security standards, ensuring that sensitive information remains protected while still being accessible to authorized users. This is crucial in environments like healthcare, where patient confidentiality is paramount, or in corporate settings where strategic information must be safeguarded.
In conclusion, while traditional search engines serve the general needs of the internet populace effectively, AI-driven, specialized search engines offer necessary capabilities that address the unique and nuanced requirements of the professional field, ensuring that sensitive, in-depth, and context-specific content is accessible with precision and security.
Introduction to AI-Powered Search Engines
AI search engine represent a significant evolution in the way information is retrieved, moving beyond traditional keyword-based search methodologies to more nuanced and intelligent systems. These advanced search platforms leverage a variety of AI technologies to interpret, predict, and deliver results that are not only relevant but also contextually aligned with the user's intent.
Definition and Core Technologies
At its core, an AI-powered search engine utilizes artificial intelligence to enhance and refine the search process. This involves several key technologies:
- Machine Learning (ML): Machine learning algorithms allow the search engine to learn from data without being explicitly programmed. By processing large amounts of queries and interactions, ML models continuously improve, learning to generate better search results based on patterns and past user behaviors.
- Natural Language Processing (NLP): NLP is crucial for understanding and processing human language. This technology helps the search engine grasp the semantics of a query, including the user’s intent and the contextual meaning of terms, which goes beyond simple keyword matching.
- Semantic Understanding: This involves interpreting the deeper meanings and relationships between words and phrases within specific contexts. Semantic technology enables the search engine to understand the query not just at a superficial level but in the way a human might understand a complex question.
These technologies work together to create a powerful system that understands and interacts in a more human-like manner.
How AI Enhances Search Capabilities
AI enhances search capabilities through several advanced functionalities:
- Aggregating and Distilling Information: AI models can process and digest multiple documents simultaneously, distilling only the most useful information. They provide users with concise answers while also offering links to the original sources, allowing users to verify and explore further. This functionality significantly enhances the efficiency of information retrieval and ensures that users have access to both summarized insights and detailed references. The human-computer interaction thus begins to resemble a human-to-human conversation, where the AI acts almost like a knowledgeable assistant.
- Understanding Query Intent: Unlike traditional search engines that rely heavily on keywords, AI-driven engines can interpret the intent behind a user’s query. For instance, when a user types "Apple," the AI can use context clues from past interactions or the user’s profile to determine whether they are referring to the fruit or the technology company.
- Improving Accuracy and Relevance: AI search engines use machine learning to refine their algorithms continuously, which means they get better at providing accurate and relevant results over time. They can analyze the relationships between different concepts, adjust based on user feedback, and learn from new data to enhance search precision.
- Personalized Search Experience: AI can tailor search results to individual users based on their search history, preferences, and even their location. This personalization ensures that users receive information that is not only accurate but also aligned with their specific needs and interests.
- Contextual and Conversational Interaction: AI search engines can engage in a more conversational and interactive manner with users. For example, they can ask follow-up questions to clarify a user’s query or offer related topics or suggestions that enrich the user’s search experience.
- Leveraging Knowledge Graphs: They map out relationships and connections between various entities and concepts in a structured form. By utilizing knowledge graphs, AI search engines can understand complex queries and the relationships between different pieces of information more deeply. For example, if a legal professional is searching for case precedents related to corporate bankruptcy, the AI can quickly pull connected concepts, such as asset liquidation, creditor priorities, or relevant bankruptcy laws, from the knowledge graph to provide comprehensive and contextually relevant information. This capability not only speeds up the retrieval of related information but also enriches the quality of the results by ensuring all related concepts are considered. By incorporating such interconnected data, AI search engines in the legal field offer a more nuanced understanding of case law and statutes, significantly aiding legal research and ensuring more informed decision-making.
These enhanced capabilities signify a shift towards more dynamic, intelligent, and user-focused search technologies that can serve a wide range of personal, professional, and academic needs more effectively than ever before. AI search engines are not just tools for finding information. They are active partners in the exploration and discovery process, capable of adapting and evolving to meet the sophisticated demands of modern users.
Challenges in Implementing AI Search Technologies
Implementing AI in search engines offers transformative potential across various fields, but it also brings significant challenges, particularly in the areas of data security, privacy, bias, and ethical considerations. Addressing these challenges is crucial for building trust and ensuring the responsible use of AI in search technologies.
Data Security and Privacy
One of the primary concerns with integrating AI into search engines is the security and privacy of data. AI systems often require access to vast amounts of data, including sensitive or personal information, to train and refine their algorithms. This raises several important questions:
- Data Vulnerability: The storage and processing of large datasets can make AI systems targets for cyber attacks. Ensuring that data is encrypted, securely stored, and accessed only by authorized systems and personnel is critical.
- Privacy Compliance: AI search engines must comply with international data protection regulations, such as GDPR in Europe or CCPA in California, which govern the use of personal data. These regulations require that data is used transparently, fairly, and with the consent of the individual.
- Ethical Data Use: Beyond legal compliance, there is a moral responsibility to use data ethically. This includes respecting user privacy and being transparent about how data is collected, used, and shared.
Developers and operators of AI search engines need to implement robust security measures and privacy-preserving techniques like data anonymization and secure data access protocols. Furthermore, ongoing monitoring and auditing are necessary to maintain security standards and user trust.
Custom GPTs and generative chatbots
In exploring the challenges associated with implementing technologies like custom GPTs by OpenAI for corporate document handling, several key issues arise that can hinder their effectiveness.
Firstly, each company maintains a unique set of documents with diverse formatting styles, making it difficult to establish a consistent content structure. For instance, distinguishing between stylistic choices like bolded text for emphasis versus new paragraph demarcations remains problematic. Additionally, the complexity of interpreting non-textual data such as tables, photos, and scanned images further complicates document processing.
Another significant challenge is the occurrence of "hallucinations", a term used to describe when AI generates incorrect or misleading information. This can lead to uncertainty about the reliability of the outputs, as the GPT might introduce content inaccurately or omit critical details. This issue is exacerbated by the AI's propensity to generate verbose content, which might not always align with the precision required in corporate settings.
Furthermore, the deployment of custom GPTs often fail to adequately reflect a company's brand voice and identity, which is crucial for maintaining consistency in corporate communications.
Issues such as AI "jailbreaks", where the model deviates from expected operational parameters, and the variability in responses to identical queries add layers of unpredictability.
Additionally, the financial burden of scaling these solutions can become prohibitive, as continuous training and customization to meet specific organizational needs require substantial investment.
Collectively, these factors present considerable obstacles to the seamless integration and practical utility of custom GPTs in corporate environments.
Conclusion: The Evolving Landscape of AI Search
In conclusion, while traditional search engines have served us well, the future of search lies in AI-driven technologies that promise more personalized, accurate, and context-aware search experiences.
As these technologies evolve, they will increasingly become integral partners in our quest for information, capable of meeting the sophisticated demands of today’s digital users. The shift to AI-powered search engines represents not just a technological upgrade, but a fundamental change in how we interact with and process the vast amounts of information available online.